Artificial intelligence (AI) has emerged as a transformative force, redefining the possibilities of what machines can achieve. However, in the conversation surrounding generative AI, it’s not uncommon to encounter a bit of confusion. People often discuss it as if it’s a whole new domain of artificial intelligence, distinct from the already sophisticated world of deep learning. Let’s go through a brief overview of the evolutionary journey of artificial intelligence to better understand how we got here.
The evolution of AI
The genesis of AI can be traced back to the mid-20th century when pioneers like Alan Turing laid the theoretical groundwork for machines altering their instructions to achieve a certain goal. Over the years, AI has evolved from rule-based systems to traditional machine-learning algorithms, finding practical applications in various domains. However, it wasn’t until the 2010s that the field experienced a paradigm shift with the take-off of deep learning.
Deep learning powered remarkable advancements for years. However, the emergence of generative AI in 2017, with the publication of the paper “Attention is All You Need” by Vaswani et al., revolutionised the field of deep learning – particularly in natural language processing tasks. This research work introduced the Transformer architecture, whose attention mechanism enabled better capture of contextual information and dependencies between different words in a sentence.
Fast forward to today, generative models have taken centre stage, especially with models like Gemini and GPT-4, which have sparked a new wave of enthusiasm and curiosity. This excitement sometimes leads to fear or misconceptions, with some assuming that generative AI is an entirely separate entity from the deep learning techniques driving AI progress. It’s essential to recognise that deep learning is, in fact, a foundational element of generative AI. To help clarify some concepts, we have created a table comparing the different AI approaches.
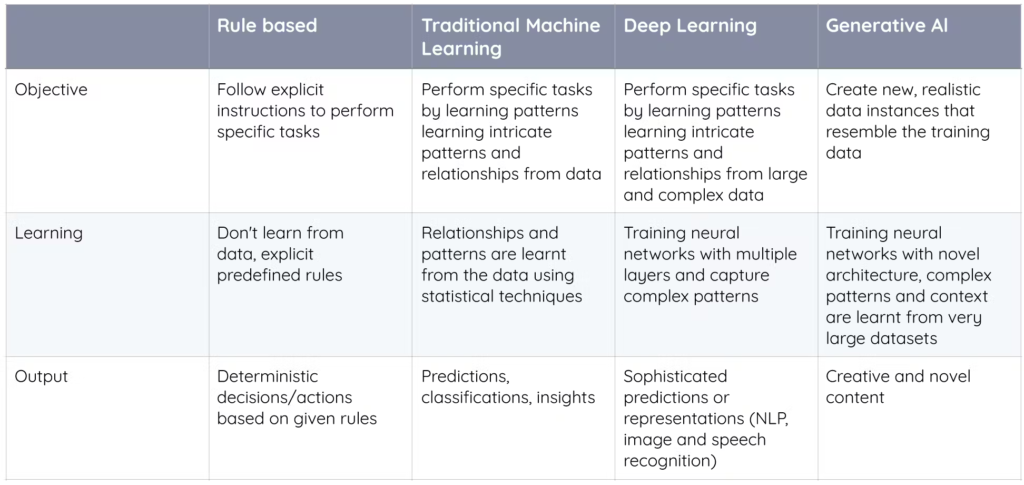
AI and data protection
Regarding our industry, AI has had a transformative impact on data protection. To illustrate this, let’s examine its influence on two real-world applications: malware detection and anomaly detection.
Malware detection
Malware detection is the process of identifying, preventing, and mitigating malicious software, commonly known as malware, within a computer system or network.
In the past, the primary defence against malware was to rely heavily on rule-based systems. These systems employed signature-based approaches, scanning for known patterns of malicious code. However, this approach faced considerable limitations when confronted with novel threats that lacked predefined signatures. The cybersecurity paradigm was in dire need of a more adaptive and intelligent solution.
Enter traditional AI, marking a pivotal shift in the approach to malware detection. Heuristic analysis and machine learning techniques took centre stage, aiming to enhance the identification of both known and unknown threats. While these methods represented a significant leap forward, challenges persisted, particularly in addressing the escalating complexity of modern malware. The quest for a more sophisticated and dynamic solution continued.
The breakthrough came with the advent of deep learning, introducing advanced technologies such as convolutional and recurrent neural networks to the realm of malware detection. This transformative phase allowed systems to automatically learn intricate features, enabling the identification of complex and evolving malware variants. Deep learning not only significantly reduced false positives, but also demonstrated a remarkable capacity to adapt to emerging threats in real time.
In the latest strides of innovation, generative AI has taken the lead in further enhancing malware detection capabilities. Generative models are being deployed to simulate potential new malware variants and to automate the generation of rules to identify malware signatures. This approach contributes to the adaptability and effectiveness of security measures, providing a robust defence.
Anomaly detection
Anomaly detection is the process of identifying unusual events or items in a data set that do not follow the normal pattern of behaviour.
Similar to malware detection, early anomaly detection relied on rule-based systems, setting predefined thresholds for normal behaviour. However, these systems struggled with the complexity and variability of real-world data. Heuristic methods and traditional machine learning algorithms were applied to anomaly detection, but while adaptive, they still faced challenges in dynamic data environments.
The advent of deep learning meaningfully improved anomaly detection. Deep neural networks, such as autoencoders (artificial neural networks that learn to compress and then reconstruct data), excelled at learning complex data patterns, enabling the identification of subtle anomalies that traditional methods might miss. In addition, generative AI – particularly generative models – found application in anomaly detection by simulating normal data distributions. This approach enhanced the adaptability of anomaly detection systems and improved the robustness of models.
What does the future hold?
While we navigate the evolving landscape of AI, both challenges and opportunities appear on the horizon. The latest generative large language models show potential in various data protection applications, such as content moderation, data anonymisation, and even automatic compliance reporting.
Balancing these capabilities with the safeguarding of sensitive data presents notable obstacles. Fortunately, there are precautionary measures we can take, such as:
- Employing hybrid methods that combine generative models with traditional rule-based systems for processing sensitive documents. This allows generative models to provide context-aware responses, while rule-based systems enforce specific constraints for compliance.
- Establishing ethical AI governance through clear guidelines and policies, regular audits, and oversight. This will be crucial to ensuring ethical AI use, particularly in handling sensitive data.
- Providing user education and obtaining explicit consent. Transparent communication about data usage will play a vital role in building trust in AI applications.
- Staying informed about changes to data protection regulations, and collaborating with legal experts to ensure that AI applications are compliant.
Implementing recommendations like these will be imperative for the responsible development and deployment of AI technologies.
So, what is the next AI milestone? This is a challenging question to answer, as advancements in the field are influenced by a multitude of factors.
For instance, do more parameters in a model equal better performance? The relationship between the number of parameters in AI models and their performance is an ongoing area of exploration.
Will we run out of data to train these models? As AI systems demand vast amounts of data for training and fine-tuning, the availability of diverse and high-quality data sets becomes critical.
Can we build artificial general intelligence (AGI)? What risks and benefits would it have?
As we venture into a future with AI, staying vigilant, adaptive, and ethically conscious will be essential.